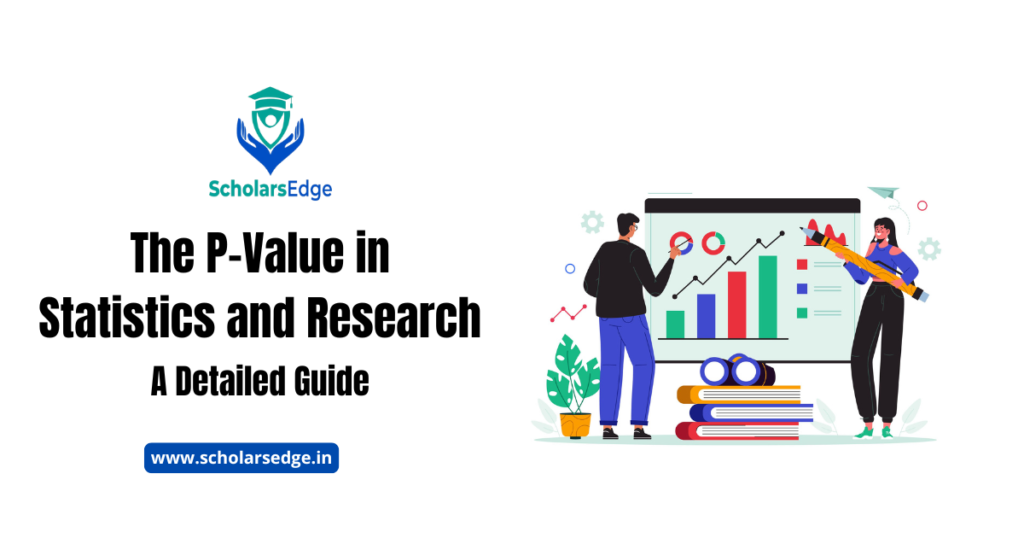
The P-value is a fundamental concept in statistical analysis, providing a measure of the probability that an observed result occurred by chance, assuming the null hypothesis is true. It plays a crucial role in hypothesis testing, helping researchers assess whether their findings are statistically significant. A lower P-value suggests more substantial evidence to reject the null hypothesis in research, guiding decision-making across fields like biostatistics, economics, and social sciences. Understanding the P-value is essential for interpreting data and making informed conclusions in any rigorous scientific study.
1. What is P-Value in Statistics?
The P-value, or probability value, quantifies the likelihood of obtaining results as extreme as those observed, assuming the null hypothesis is correct. In essence, it helps evaluate whether the observed data fits within the expectations of the null hypothesis.
In hypothesis testing, the P-value is compared to a pre-determined significance level (α), often set at 0.05. If the P-value is smaller than α, the result is considered statistically significant, and the null hypothesis is rejected. The significance level (α) represents the threshold for deciding if the evidence is strong enough to reject the null hypothesis. Unlike the P-value, α is fixed before the study begins and reflects the researcher’s tolerance for false positives (Type I errors).
2. P-Value in Biostatistics and Research
Biostatistics widely uses the P-value to evaluate medical research, clinical trials, and epidemiological studies. It aids in determining whether a treatment effect or association is statistically significant and not just due to random variation.
For researchers, the P-value offers a standardized method to quantify the strength of the evidence against the null hypothesis. In fields like public health, where the interpretation of data can affect policies, treatments, and patient outcomes, understanding the P-value is crucial. A well-interpreted P-value can guide decisions about the effectiveness of interventions, treatments, or potential risk factors in population studies.
3. What Does the P-value indicate?
A lower P-value, typically less than 0.05, suggests that the observed data is unlikely under the null hypothesis, indicating stronger evidence to reject the null hypothesis. This often implies that there is a statistically significant effect or association present.
Conversely, a higher P-value indicates weaker evidence against the null hypothesis, meaning the observed data could reasonably occur if the null hypothesis were true. In this case, researchers usually fail to reject the null hypothesis, as insufficient evidence supports an alternative explanation.
The P-value is essential in determining statistical significance, which informs whether findings can be generalized or should be further investigated. However, it’s important to note that the P-value doesn’t measure an effect’s size or practical importance but simply the strength of the evidence against the null hypothesis.
4. Equation for P-Value and P-Test Formula
The P-value is calculated from various test statistics depending on the type of hypothesis test being conducted, such as a t-test or z-test. While the exact calculation can vary, the general approach is consistent across tests.
The basic formula for calculating the P-value can be expressed as:
P(value)=P(test statistic∣null hypothesis is true)
Where the test statistic is typically calculated using a formula specific to the hypothesis test being applied. For example, in a t-test, the formula for the test statistic is:
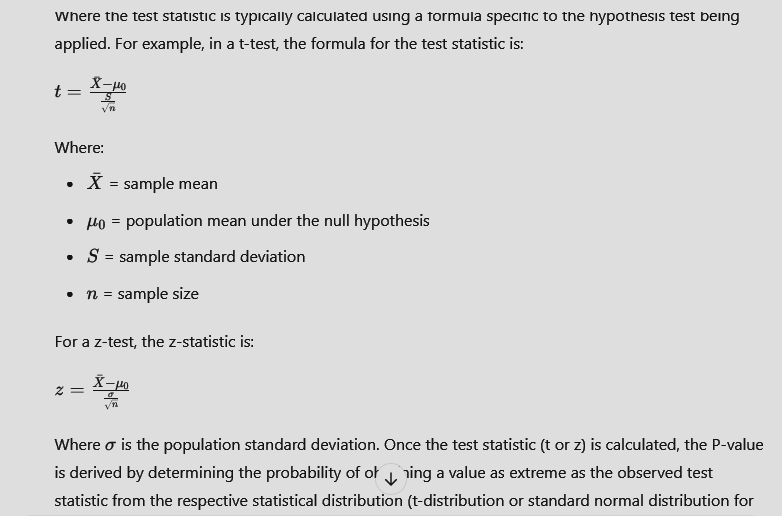
Where σ (sigma) is the population standard deviation, once the test statistic (t or z) is calculated, the P-value is derived by determining the probability of obtaining a value as extreme as the observed test statistic from the respective statistical distribution (t-distribution or standard normal distribution for z-tests).
Steps to derive the P-value from test statistics:
- Calculate the test statistic using the relevant formula.
- Identify the distribution (t-distribution or z-distribution).
- Find the probability of obtaining a test statistic as extreme as the one calculated, assuming the null hypothesis is true.
- Compare the P-value to the significance level (α) to determine if the result is statistically significant.
5. How to Calculate P-Value
Manual Calculation Process Using Statistical Formulas Manually calculating the P-value involves determining the test statistic (e.g., t or z) based on the type of test and data. Once the test statistic is found, it is compared to a standard distribution table (t-distribution for a t-test, z-distribution for a z-test) to find the probability that the result is as extreme as or more extreme than the observed value.
For example, in a two-tailed test:
- Determine the test statistic (e.g., t-statistic or z-statistic).
- Consult the appropriate distribution table (t-table or z-table) to find the corresponding probability (P-value).
- If necessary, Adjust for a two-tailed test by multiplying the one-tailed P-value by two.
While manual calculations are possible, they can be complex and time-consuming, especially for large datasets or more advanced tests. Therefore, P-value calculators are often used to streamline this process.
Overview of Online P-Value Calculators Online P-value calculators are widely available tools that allow users to input test statistics (such as t-statistic or z-statistic) and instantly calculate the corresponding P-value. These calculators save time and reduce the potential for errors in manual calculations. They are instrumental when dealing with more complex tests or large datasets.
Step-by-Step Guide to Calculating P-Value from T-Statistic and Z-Statistic
- T-Statistic Calculation:
- Perform a t-test by computing the test statistic using the earlier formula.
- Enter the t-statistic, degrees of freedom, and significance level into an online P-value calculator or use a t-distribution table.
- The calculator will provide the P-value based on the inputs.
- Z-Statistic Calculation:
- For a z-test, calculate the z-statistic using the formula.
- Use a standard normal distribution table or online calculator to convert the z-statistic to a P-value.
- For a two-tailed test, double the P-value obtained from the one-tailed test.
6. P-Value Calculators and Their Usage
Introduction to P-Value Calculators Available Online Many online platforms offer P-value calculators for free. These tools require inputs such as the test statistic, sample size, and degrees of freedom. Once entered, they return the P-value, allowing researchers to assess the significance of their results quickly.
Some popular P-value calculators include:
- GraphPad
- Social Science Statistics
- EasyCalculation.com
How to Use These Calculators Effectively To use a P-value calculator:
- Identify which test (t-test, z-test) you are using.
- Input the relevant test statistic, degrees of freedom (if applicable), and other parameters.
- Choose one-tailed or two-tailed options, depending on your hypothesis.
- The calculator will instantly provide the P-value, which can then be compared to your chosen significance level (α).
Explanation of P-Value from T-Statistic and Z-Interval A P-value derived from a t-statistic or z-interval quantifies the probability that the observed test statistic would occur under the null hypothesis. In a z-interval, the P-value is based on the normal distribution. In contrast, a t-test is based on the t-distribution, which adjusts for smaller sample sizes and greater variability.
7. Common Questions About P-Value
How Do I Get a P-Value in Research? P-values are obtained by performing statistical tests on your data. After calculating the test statistic (e.g., t or z), you can use a table or calculator to find the P-value. Many statistical software packages like R, SPSS, and Excel also provide P-values automatically when performing hypothesis tests.
What Does a Significant P-Value Mean in Practice? A significant P-value (typically less than 0.05) suggests that the observed data are unlikely to have occurred by chance alone, assuming the null hypothesis is true. In practice, there is enough evidence to reject the null hypothesis, supporting the alternative hypothesis.
Misconceptions About P-Value One common misconception is that the P-value represents the probability that the null hypothesis is true, which is not correct. The P-value indicates the likelihood of observing the data if the null hypothesis were true, not the truthfulness of the hypothesis itself.
8. P-Score and Its Relevance
Definition and Explanation of P-Score The P-score, or performance score, is often confused with the P-value but is a different metric used in various contexts, including educational testing and performance assessments. It measures how well an individual or system performs relative to a set of criteria.
Difference Between P-Value and P-Score While the P-value is a probability related to statistical significance in hypothesis testing, the P-score is used to assess performance outcomes and doesn’t play a role in hypothesis testing.
Application of P-Score in Statistical Tests In some areas of machine learning and performance evaluation, the P-score might be applied. Still, it is distinct from the P-value, tied explicitly to probability and statistical significance.
9. P-Value Interpretation in Research
How to Interpret Statistically Significant Results Based on P-Value When interpreting P-values, a result below the significance level (e.g., 0.05) suggests strong evidence against the null hypothesis. However, statistical significance does not equate to practical importance, so researchers should consider effect sizes and real-world relevance.
Examples of P-Value Interpretation in Biostatistics: a P-value might be used to determine if a new treatment is more effective than a placebo. If the P-value is below 0.05, researchers would conclude that the treatment has a statistically significant effect.
Real-World Scenarios Where P-Value Played a Crucial Role in Research P-values are crucial in clinical trials, where they help decide whether a new drug should be approved or if a particular medical intervention is effective. For instance, a low P-value in a trial might lead to the approval of a life-saving medication.
Conclusion
Understanding the P-value is essential for interpreting statistical results and making informed decisions in research. Whether calculating it manually or using a calculator, the P-value helps assess the strength of evidence against the null hypothesis. While a significant P-value can indicate statistical significance, it’s crucial to interpret it alongside effect sizes and practical relevance to draw meaningful conclusions in scientific studies.
FAQs
What is P-value in research statistics?
In research statistics, the P-value measures the probability of obtaining the observed results or something more extreme, assuming the null hypothesis is true. It helps researchers assess whether their findings are stat
istically significant. A smaller P-value suggests stronger evidence against the null hypothesis, indicating that the observed effect is unlikely to be due to chance. This value plays a key role in hypothesis testing and decision-making in research.
What does the p-value of 0.05 mean?
A P-value of 0.05 means there is a 5% chance that the observed results occurred by random chance, assuming the null hypothesis is true. If the P-value is less than or equal to 0.05, the results are considered statistically significant, leading to the rejection of the null hypothesis. Researchers typically use 0.05 as a threshold to decide whether the data provides sufficient evidence to support their alternative hypothesis.
When to use 0.01 and 0.05 levels of significance?
The 0.05 significance level is commonly used in research when a moderate level of evidence is required to reject the null hypothesis. A 0.01 significance level is used when stronger evidence is needed, typically in high-stakes studies (e.g., medical research). A lower significance level (0.01) reduces the likelihood of false positives (Type I errors), making it more appropriate for fields where the consequences of errors are critical.