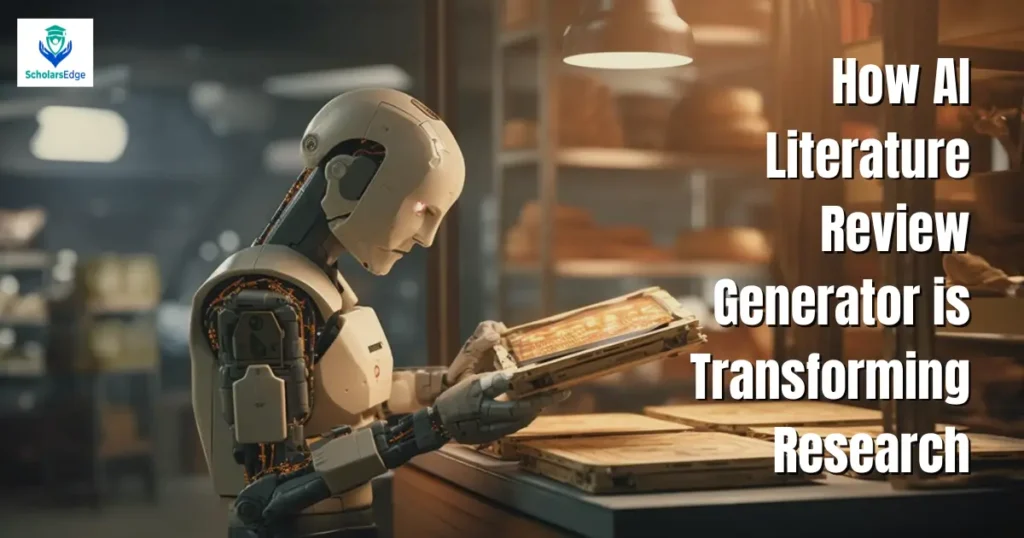
Artificial Intelligence (AI) is increasingly becoming a pivotal force in academic research, particularly in the area of literature reviews. Conducting a literature review has traditionally been a labor-intensive process, fraught with challenges such as information overload, time constraints, and the need for organization. However, with the advent of AI-powered literature review generators, researchers can now direct these challenges more efficiently and effectively. This article explores how AI literature review generators are transforming research, highlighting their benefits, popular tools, operational mechanisms, and future prospects.
Introduction
The literature review is a critical component of the research process, serving as a foundation for understanding existing knowledge and identifying gaps in the field. Researchers often need help with conducting these reviews, including the sheer volume of available literature and the complexity of synthesizing diverse sources into coherent narratives. Traditional methods require extensive manual effort to collect, analyze, and summarize relevant studies, which can be both time-consuming and prone to human error. AI offers a solution to these challenges by automating various aspects of the literature review process. AI-powered tools can streamline tasks such as searching for relevant articles, extracting key insights, and organizing findings into structured formats. As interest in these technologies grows among researchers, scholars, and academic institutions, it is essential to understand how AI is reshaping the literature reviews.
Why Use AI for Literature Review Generation?
Time-Saving Benefits
One of the most significant advantages of using AI for literature reviews is the substantial reduction in time required to conduct comprehensive reviews. AI tools can process vast amounts of data in minutes—something that would take researchers weeks or even months to accomplish manually. By automating repetitive tasks such as searching databases and filtering results based on predefined criteria, researchers can focus their efforts on interpreting findings rather than getting bogged down in data collection.
Enhanced Accuracy
AI tools enhance the accuracy of literature reviews by minimizing human error and bias. Traditional reviews are often influenced by subjective interpretations or incomplete searches, leading to potential oversights or misrepresentations of existing research. AI algorithms, trained on large datasets, can objectively analyze literature, ensuring that relevant studies are included while filtering out irrelevant or low-quality publications.
Improved Organization
AI-powered literature review generators excel at organizing information effectively. These tools categorize studies based on themes or topics, summarize key findings, and create visual representations of connections between different pieces of research. This structured approach aids in comprehension and facilitates easier navigation through complex information.
Popular AI Tools for Literature Review Generation
Here is a table summarizing the features and benefits of the AI tools for literature reviews:
AI Tool | Key Features | Benefits |
Litmaps | – Interactive platform for visualizing literature landscapes | – Helps researchers track citations over time and map related works dynamically. |
– Dynamic citation tracking | ||
ResearchRabbit | – Automates citation management | – Facilitates the discovery of new papers related to existing references, improving research depth and coverage. |
– Integrates with popular reference management tools | – Provides seamless integration with other reference management software for easier data organization. | |
Scite.ai | – Analyzes citation context to evaluate research credibility | – Offers insights into how studies are cited, highlighting whether papers support or contradict previous findings. |
– Assists in quality assessment by showing citation context | – Enhances the quality of literature reviews by allowing users to assess the reliability and impact of sources. |
These tools offer unique features that cater to different aspects of the literature review process, from citation tracking to concept mapping.
How AI Literature Review Generators Work
Data Collection and Processing
AI literature review generators gather data from various academic sources such as PubMed, Google Scholar, and institutional repositories. They employ web scraping techniques and APIs to access vast databases of scholarly articles efficiently.
Text Summarization Algorithms
Once data is collected, AI tools utilize text summarization algorithms to condense lengthy articles into digestible summaries. These algorithms extract key phrases and insights while preserving essential information about methodologies and findings.
Keyword Extraction and Concept Mapping
AI plays a crucial role in identifying core themes through keyword extraction techniques. By analyzing text patterns and frequency of terms across multiple studies, AI can map out concepts that are central to specific research areas.
Natural Language Processing (NLP)
NLP is at the heart of many AI literature review tools. It enables machines to understand human language nuances, allowing them to process complex academic texts effectively. NLP algorithms facilitate tasks such as sentiment analysis and context understanding, further enhancing the quality of generated summaries.
Types of Literature Reviews AI Can Generate
AI is capable of generating various types of literature reviews:
- Systematic Reviews: These comprehensive reviews follow strict protocols to identify all relevant studies on a particular topic. AI can assist by automating search strategies and data extraction processes.
- Narrative Reviews: For broader topics that require cohesive storytelling rather than strict methodology adherence, AI can generate narrative reviews by synthesizing information across diverse studies into a unified narrative.
- Scoping Reviews and Meta-Analysis: Certain tools facilitate scoping reviews by identifying gaps in existing research, while meta-analysis tools assist in quantitatively synthesizing data from multiple studies.
Future of AI in Literature Review Generation
Integration with Research Workflows
The future promises deeper integration between AI tools and existing research workflows. As academic institutions increasingly adopt these technologies, we can expect smoother transitions between stages of research—from initial literature searches to final manuscript preparation.
Advancements in Generative AI
Generative models like GPT-4 are shaping how we approach literature synthesis by providing advanced capabilities for summarization and content generation. These models could further enhance the depth and breadth of insights generated during literature reviews.
Potential for Personalized Literature Reviews
In the future, personalized literature review experiences may become commonplace. By leveraging individual researcher profiles—such as previous works or specific interests—AI could tailor recommendations and summaries that align closely with each researcher’s unique needs.
Conclusion
The transformative role of AI in literature review generation cannot be overstated. By automating time-consuming processes while enhancing accuracy and organization, AI tools empower researchers to conduct thorough reviews more efficiently than ever before. However, it is crucial to recognize that these technologies should complement—not replace—human expertise in research. As researchers continue to explore the capabilities offered by AI tools, they are encouraged to experiment with these innovations to enhance their own literature review processes. The combination of advanced technology with critical human insight holds great promise for advancing knowledge across disciplines. Embracing this synergy will not only streamline research efforts but also foster deeper understanding within academic communities worldwide.
FAQs
How does AI reduce human bias in literature reviews?
AI significantly reduces human bias in literature reviews by automating the selection and analysis processes, which minimizes subjective interpretations that can skew results. Traditional literature reviews often rely on individual researchers’ judgments, which can be influenced by personal biases or incomplete knowledge. AI tools, however, utilize algorithms that analyze vast datasets objectively, ensuring that all relevant literature is considered based on predefined criteria rather than personal preference. These algorithms can also identify and flag potential biases in the literature itself, providing a more balanced view of existing research. Consequently, AI enhances the integrity and reliability of literature reviews by promoting a more systematic and impartial approach to data analysis.
What are the unique features of popular AI tools like Litmaps and ResearchRabbit?
Popular AI tools like Litmaps and ResearchRabbit offer unique features tailored to streamline the literature review process. Litmaps provides an interactive visualization of research connections, allowing users to track citations over time and discover related works through dynamic mapping. This feature helps researchers see how studies are interconnected, facilitating a deeper understanding of the field. On the other hand, ResearchRabbit automates citation management by integrating with popular reference management software such as Mendeley and Zotero. It simplifies the process of importing and organizing references while also suggesting new papers based on existing citations.