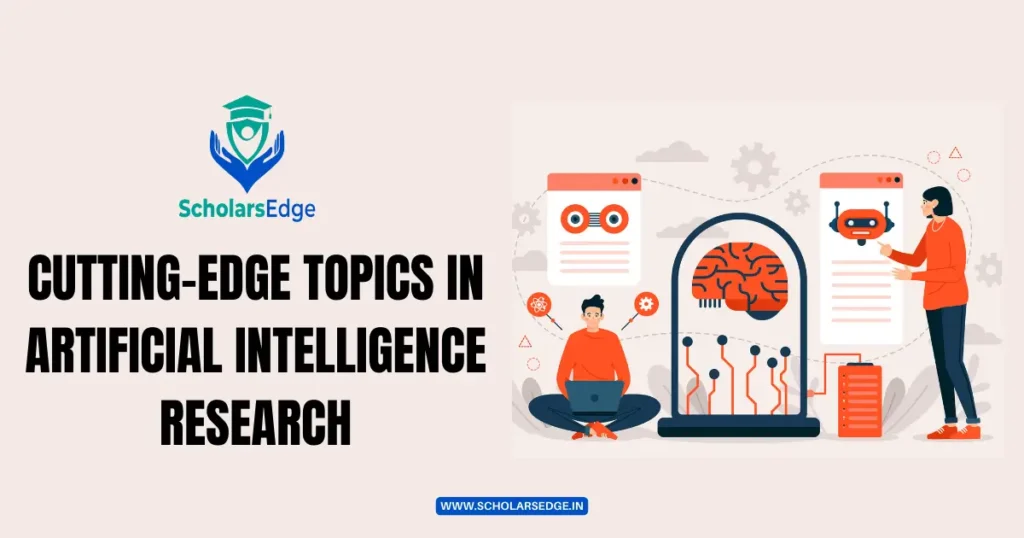
Artificial Intelligence (AI) has evolved from a speculative concept to a transformative force impacting various industries and aspects of daily life. Its significance lies in its ability to solve complex problems, enhance efficiency, and drive innovation across diverse sectors. For Ph.D. research scholars, professors, and researchers, staying updated with the latest trends in AI research is crucial for contributing to and leveraging these advancements.
List of 25 cutting-edge topics in artificial intelligence research:
No. | Topic | Description |
1 | Explainable AI (XAI) | Developing AI models that are more transparent and provide understandable explanations for their decisions. |
2 | AI Ethics and Fairness | Ensuring AI systems are developed and deployed in an ethical, unbiased, and fair manner. |
3 | Generative AI | Using machine learning to create new data like images, text, and audio that resembles input data. |
4 | Conversational AI | We are advancing natural language processing techniques to enable more natural, human-like interactions with AI assistants. |
5 | Reinforcement Learning | Developing algorithms that allow AI agents to learn optimal behaviours by interacting with dynamic environments. |
6 | Federated Learning | Training AI models collaboratively using decentralised data without sharing the raw data. |
7 | Quantum Computing for AI | Exploring how quantum computing can enhance and accelerate AI algorithms. |
8 | Neuromorphic Computing | We are developing hardware inspired by the human brain to enable more efficient and brain-like AI systems. |
9 | Adversarial Machine Learning | It is improving the robustness of AI models against adversarial attacks that try to fool the system. |
10 | Automated Machine Learning (AutoML) | It is creating systems that can automatically design and optimise machine learning models. |
11 | Continual/Lifelong Learning | We are enabling AI agents to continuously learn and adapt without forgetting previous knowledge. |
12 | Multimodal Learning | Developing AI models that can process and integrate information from multiple sensory modalities. |
13 | Swarm Intelligence | Designing decentralised, self-organising systems inspired by the collective behaviour of social insects. |
14 | Embodied AI | Studying how physical embodiment and interaction with the environment can improve AI capabilities. |
15 | Causal Reasoning | Advancing AI’s ability to understand and reason about cause-and-effect relationships. |
16 | Neuro-Symbolic AI | Combining neural networks with symbolic reasoning to create more interpretable and compositional AI. |
17 | Trustworthy AI | Ensuring AI systems are reliable, secure, and aligned with human values. |
18 | AI for Sustainability | Applying AI to address environmental challenges like climate change, resource management, and biodiversity conservation. |
19 | AI for Healthcare | Developing AI-powered tools for disease diagnosis, drug discovery, personalised treatment, and patient monitoring. |
20 | AI for Education | Leveraging AI to create personalised learning experiences, intelligent tutoring systems, and adaptive assessments. |
21 | AI for Scientific Discovery | Using AI to accelerate scientific research, generate hypotheses, and uncover new scientific insights. |
22 | AI for Creative Expression | Exploring the use of AI in generating art, music, literature, and other creative content. |
23 | AI Safety | Ensuring the safe and controlled development of advanced AI systems to mitigate potential risks. |
24 | AI Governance | Establishing policies, regulations, and frameworks to guide the responsible development and deployment of AI. |
25 | AI-Human Interaction | Improving the ways humans and AI systems can collaborate, communicate, and work together effectively. |
Natural Language Processing (NLP)
Natural Language Processing (NLP) is a subfield of AI focused on the interaction between computers and human languages. It enables machines to understand, interpret, and generate human language, making it indispensable in various applications. NLP applications are widespread, including chatbots, sentiment analysis, language translation, and more. Tools like ChatGPT and Bard have brought NLP into the mainstream, demonstrating its potential in creating human-like text and facilitating natural conversations.
Key Research Areas
- Language Generation: This involves creating systems capable of generating coherent and contextually relevant text. Advances in transformer models, such as GPT-3, have set new benchmarks in this area.
- Sentiment Analysis: This area focuses on extracting and understanding emotions from text data, which is crucial for applications in marketing, customer service, and social media monitoring.
- Machine Translation: Developing models that accurately translate text from one language to another remains a significant focus, with neural machine translation systems leading the charge.
Computer Vision
Computer Vision (CV) enables machines to interpret and process visual data, simulating the human vision system. It plays a pivotal role in various sectors, from healthcare to autonomous driving. Computer vision technologies are used in image and video analysis, object detection, facial recognition, and more. For instance, in healthcare, computer vision aids in medical imaging, enhancing diagnostic accuracy.
Research Topics
- Image Recognition: This involves identifying objects or features within an image, a fundamental capability for many AI applications.
- Object Detection: This area goes beyond recognising objects and focuses on accurately detecting and localising them within an image or video frame.
- Video Analysis: Analyzing video data in real-time for applications like surveillance, sports analytics, and autonomous vehicles is a rapidly growing research area.
Reinforcement Learning
Reinforcement Learning (RL) is a type of machine learning in which agents learn to make decisions by interacting with their environment. It has seen significant advancements and applications in various fields. RL involves agents taking actions to maximise cumulative rewards. This approach is essential for developing systems that can learn and adapt over time, such as autonomous robots and game-playing AI.
Key Topics
- Deep Reinforcement Learning: Combining deep learning with RL algorithms has led to breakthroughs in areas such as game playing (e.g., AlphaGo) and robotics.
- Multi-Agent Systems: Research in this area focuses on how multiple agents can collaborate or compete, which is vital for applications in traffic management and strategic games.
- Applications in Robotics: RL is crucial for developing robots that can learn complex tasks through trial and error, enhancing their autonomy and efficiency.
Ethics and AI
As AI systems become more pervasive, addressing ethical considerations is paramount. Ethical AI ensures that these systems are developed and deployed responsibly. Ethical AI addresses issues such as bias, transparency, and governance. Ensuring that AI systems are fair, transparent, and accountable is critical for maintaining public trust and preventing harm.
Research Areas
- Bias Mitigation: Developing methods to identify and mitigate biases in AI systems to ensure fair outcomes for all users.
- Transparency: Creating AI models and systems that are explainable and understandable to users and stakeholders.
- AI Governance: Establishing frameworks and policies to govern the ethical development and deployment of AI technologies.
AI in Healthcare
AI’s applications in healthcare are revolutionising medical diagnosis, treatment, and patient care. By leveraging AI, healthcare systems can become more predictive, personalised, and efficient.
Applications in Medical Diagnosis and Treatment
AI tools diagnose diseases, predict patient outcomes, and personalise treatment plans. For instance, AI-driven predictive analytics can forecast disease outbreaks, while personalised medicine tailors treatments to individual patients based on their unique genetic makeup.
Research Topics
- Predictive Analytics: Using AI to predict health trends, patient outcomes, and potential epidemics.
- Personalized Medicine: Developing AI systems that analyse individual genetic profiles to provide tailored treatment plans.
- Medical Imaging: Enhancing the accuracy and efficiency of interpreting medical images through AI, leading to better diagnostic capabilities.
Conclusion
Artificial Intelligence continues to advance rapidly, with significant implications for various fields. Staying abreast of cutting-edge topics such as NLP, computer vision, reinforcement learning, ethics in AI, and AI in healthcare is essential for researchers and academics. By exploring these areas, scholars can contribute to the development of innovative solutions and ensure that AI technologies are used responsibly and effectively.
For researchers, delving into these topics offers the opportunity to push the boundaries of what AI can achieve, driving forward both academic inquiry and practical applications.
FAQs About Topics in Artificial Intelligence Research
Which topic is best for research in AI?
Based on current research trends, the best topics for AI research include developing new machine learning algorithms and models, especially in supervised, unsupervised, and reinforcement learning, advancing deep neural network architectures for applications like computer vision, natural language processing, and speech recognition, improving language understanding and generation in NLP, enhancing techniques in computer vision for image and video analysis, creating intelligent autonomous systems in robotics, making AI models more transparent and explainable, ensuring AI is developed and deployed ethically and fairly, and applying AI solutions to specific domains such as healthcare, sustainability, cybersecurity, and finance. These areas not only drive technological progress but also address critical ethical and practical considerations, ensuring AI technologies are both powerful and responsible.
What are the most promising AI topics for future research?
The most promising AI research topics for the future include advancing machine learning and deep learning algorithms and models, especially in areas like natural language processing, computer vision, and robotics; developing explainable AI systems that provide transparent and understandable decisions; ensuring AI is developed and deployed ethically and without bias; applying AI to critical domains like healthcare, sustainability, and cybersecurity; exploring the potential of quantum computing and cloud computing to enhance AI; and investigating computational intelligence inspired by natural intelligence. The key focus is on pushing the boundaries of AI capabilities while prioritising safety, fairness, and beneficial applications.
What is the main area of research in AI?
The main area of research in AI currently focuses on several key fields, reflecting the dynamic advancements and diverse applications of this technology. Generative AI, which involves creating new content such as text, images, and videos using AI models, is a prominent research focus due to its rapid adoption and significant impact across industries. Machine learning, particularly deep learning, remains fundamental, driving progress in computer vision, natural language processing, and multimodal models that handle multiple types of data simultaneously. Additionally, the integration of AI in robotics to develop more flexible and autonomous systems is a critical area of exploration. Another vital research domain is responsible AI, which encompasses efforts to ensure AI systems are ethical, transparent, and fair, addressing issues like bias mitigation and explainability. As AI continues to evolve, these areas not only push the boundaries of what is technologically possible but also ensure that AI advancements are implemented responsibly and effectively.